From the desk of Thiago Passos, CEO at April9:
I was recently fortunate enough to attend the Queensland AI Summit, and got to hear some very intelligent humans talk about their very intelligent robots. Presenters ranged from engineers to business leaders, all experts in the AI field, including guests from MIT, Google, and more.
One talk that really fascinated me was by Daniela Rus – Director of MIT's Computer Science and Artificial Intelligence Lab - regarding Liquid Neural Networks, and the potential use cases and implications for AI. I wanted to share the key takeaways from her presentation here since I think it’s not only fascinating but also highly relevant to the way that AI implementation may be evolving.
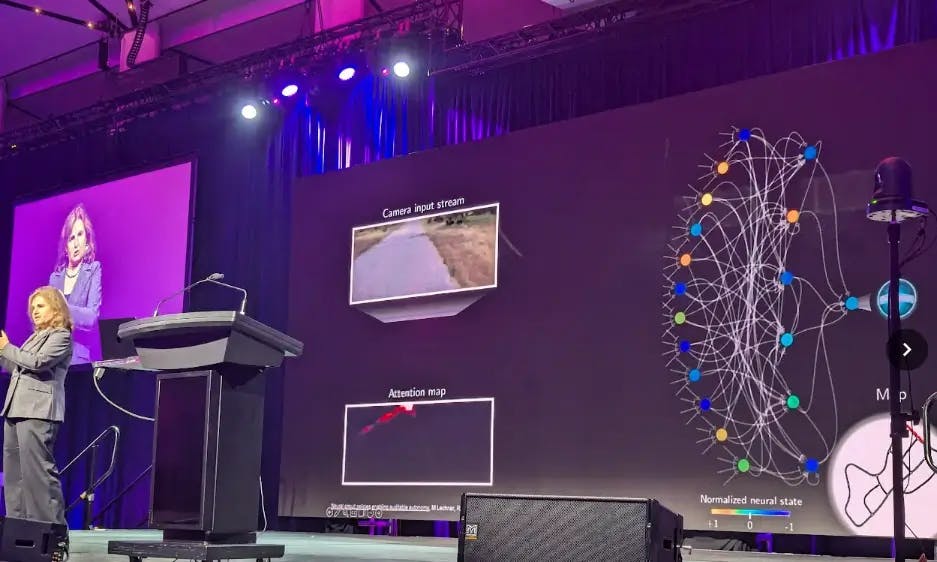
@ the Queensland AI Summit 2024
A Quick Refresher For Anyone Living Under A Rock
Artificial intelligence (AI) has transformed industries, bringing advancements in automation, data analysis, and creative pursuits. Central to these developments are AI foundation models (FMs)—powerful, large-scale models capable of performing different tasks without needing extensive fine-tuning.
Yet, as the demand for more intelligent, adaptive, and context-aware AI increases, researchers are exploring innovative architectures to take AI to the next level. Unfortunately, AI’s rigidity and computational costs often hinder traditional neural networks, making model adaptability difficult.
But newer concepts are gaining traction, and one such concept is the liquid network, which promises to revolutionise AI foundation models by making them more adaptable, dynamic, and efficient. Here is what you need to know about the fusion of liquid networks and AI.
Understanding Liquid Neural Networks
A liquid network is a novel approach to neural network design, inspired by neuroscience principles and the brain’s ability to adapt dynamically to new information.
In contrast to traditional, static neural networks with fixed weights and structures, liquid networks can adjust their parameters on the fly based on incoming data. This adaptability enables them to process information more fluidly, “learning” and evolving without retraining or significant adjustments.
Liquid networks have non-linear, time-dependent properties, which allow them to capture the complex patterns inherent in dynamic environments.
The concept was introduced by the Massachusetts Institute of Technology (MIT) researchers, who drew inspiration from liquid brain structures in certain animals. These structures allow for real-time adaptation, as the animals can reconfigure their neural pathways in response to environmental changes.
Similarly, a liquid network updates its architecture and weight distributions on the fly, enabling it to respond to new situations.
How Liquid Networks Differ from Traditional AI Models
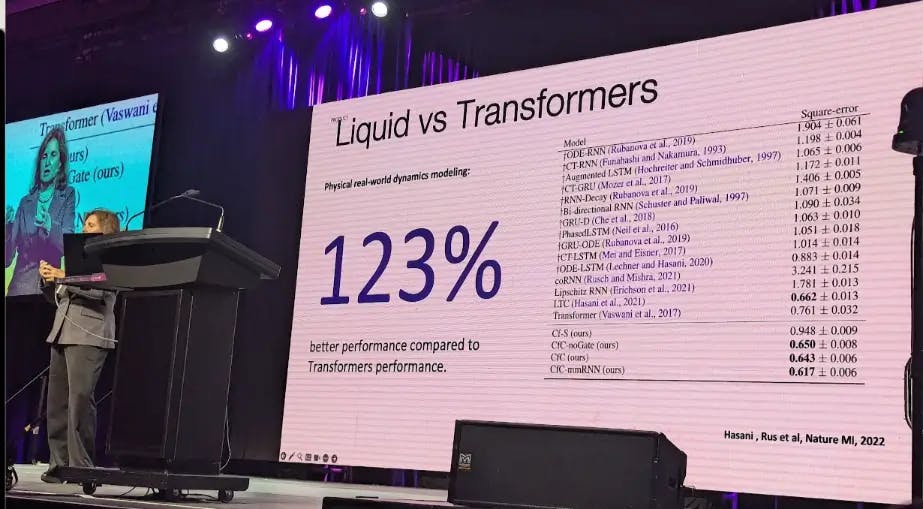
LNNs vs Transformers
Traditional neural networks, such as convolutional neural networks (CNNs) and recurrent neural networks (RNNs), operate on fixed structures.
Once trained, their parameters (or weights) are “locked in,” meaning they can’t adapt dynamically without retraining. While this approach is effective for stable and predictable environments, it becomes inefficient and impractical in scenarios requiring rapid adaptation or real-time decision-making.
On the other hand, liquid networks have flexible structures that allow them to adapt as they process data. This is achieved by utilising differential equations that change over time based on the input they receive. The adaptability of these networks is similar to that of human cognition, allowing them to maintain performance and accuracy in unpredictable or unfamiliar situations.
Essentially, liquid networks could enable AI models to operate in dynamic environments, such as autonomous vehicles, real-time health monitoring systems, or volatile financial markets.
Revolutionising AI Foundation Models with Liquid Networks
AI foundation models, such as GPT-4, BERT, and DALL-E, can handle vast amounts of data and perform multiple tasks accurately. However, these models have limitations with adaptability and real-time responsiveness.
Liquid networks offer the potential to revolutionise foundation models by addressing these limitations in several key ways:
1. Improved Adaptability and Real-Time Learning
Liquid networks could enable AI foundation models to adapt their behaviour in real time. Imagine a language model that can adjust its tone and response style based on user feedback without further training.
With liquid networks, such adaptability is achievable, as the network can dynamically adjust its parameters in response to new data. This adaptability would make AI foundation models more contextually aware, allowing them to function effectively across different applications and respond to unique situations.
2. Enhanced Memory and Context Awareness
Liquid networks offer an advantage in maintaining memory over extended periods. Traditional foundation models often struggle with retaining contextual information, especially in tasks involving long-term dependencies.
Liquid networks, with their continuous adaptability, can retain and utilise information over time, enhancing their contextual awareness. For example, a customer service AI could remember past user interactions and adjust responses based on historical data to ensure a personalised experience.
3. Greater Efficiency and Reduced Computational Costs
AI foundation models are typically resource-intensive, requiring significant computational power and storage.
Liquid networks can make these models more efficient by reducing the need for extensive retraining. As they can adapt continuously, liquid networks eliminate the need for retraining cycles, saving time, energy, and costs.
This efficiency is particularly valuable for large-scale models deployed in real-time applications, such as autonomous systems or streaming analytics, where frequent retraining would be impractical.
4. Enhanced Robustness in Unpredictable Environments
In dynamic and unpredictable environments, AI models need to make split-second decisions while adapting to constantly changing conditions. Liquid networks provide the foundation models with this level of robustness.
In fields like healthcare, for instance, an AI model powered by a liquid network could dynamically adjust its recommendations based on real-time patient data, accommodating sudden changes in health status. This adaptability allows AI foundation models to perform effectively in critical, high-stakes situations.
5. Unleashing New Applications for AI
With the liquid network’s enhanced adaptability and efficiency, AI foundation models could unlock new applications that were previously challenging or impossible to implement.
For instance, they could be used in disaster response scenarios, where AI models would need to assess shifting conditions and make rapid decisions to aid rescue operations. Additionally, in industries such as finance, AI could dynamically adapt to economic shifts, offering real-time insights and analysis for more informed decision-making.
Challenges and Future Prospects

While the liquid network’s potential to revolutionise AI foundation models is significant, there are challenges to consider.
The development and implementation of liquid networks require a deep understanding of differential equations and time-varying parameters, which adds complexity to the design process. Additionally, these networks may be computationally intensive, potentially offsetting some efficiency gains.
However, as research in this field progresses, these challenges can be addressed, making liquid networks accessible to mainstream AI applications. Advances in hardware, such as quantum computing and neuromorphic chips, could further support the integration of liquid networks into large-scale AI systems.
Related reading: Digital Transformation Strategies for SMEs and IT Leaders
Deploy AI Capabilities in Your Business with April9
Liquid networks represent an exciting frontier in AI research, offering the potential to make AI foundation models more adaptive, efficient, and context-aware. By enabling real-time learning and enhanced memory capabilities, these networks could transform how AI models operate, opening up new possibilities across industries.
As AI evolves, liquid networks could reshape the future of intelligent, responsive, and versatile AI systems. At April9, we believe AI can transform business processes by handling complex processes like customer review aggregation, software testing, or even data parsing and segmentation. These solutions can work alongside humans to drive automation and increase efficiency.
Contact April9 today and learn how we can help your business deploy AI capabilities.
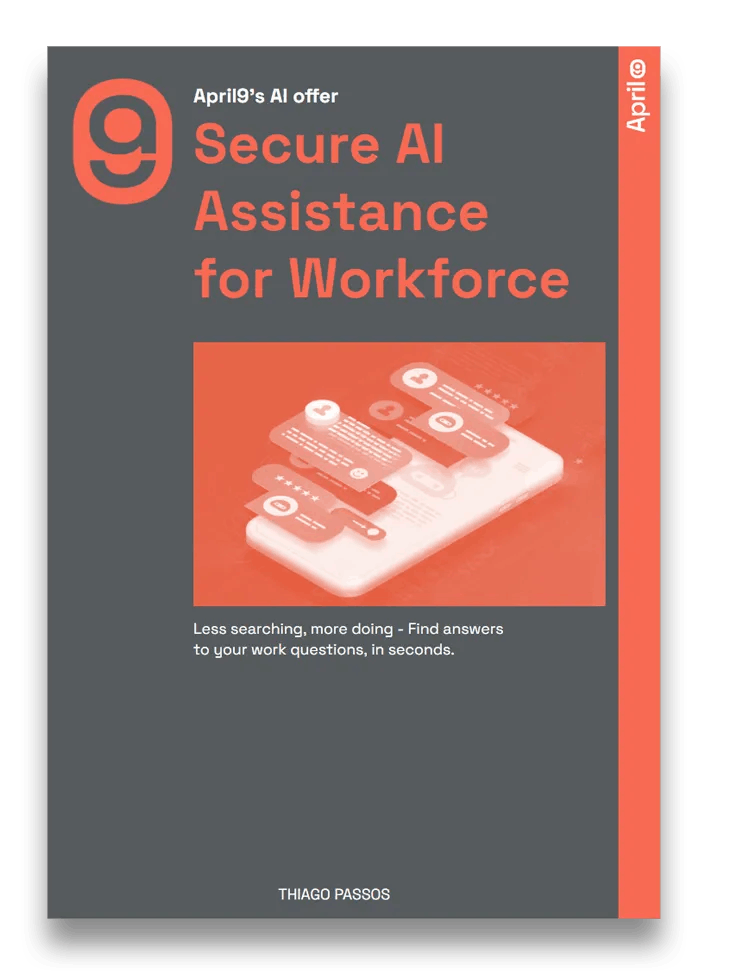
Download our free eBook “5 ways to master Data-Driven Decisions with an AI Back-Office Assistant”